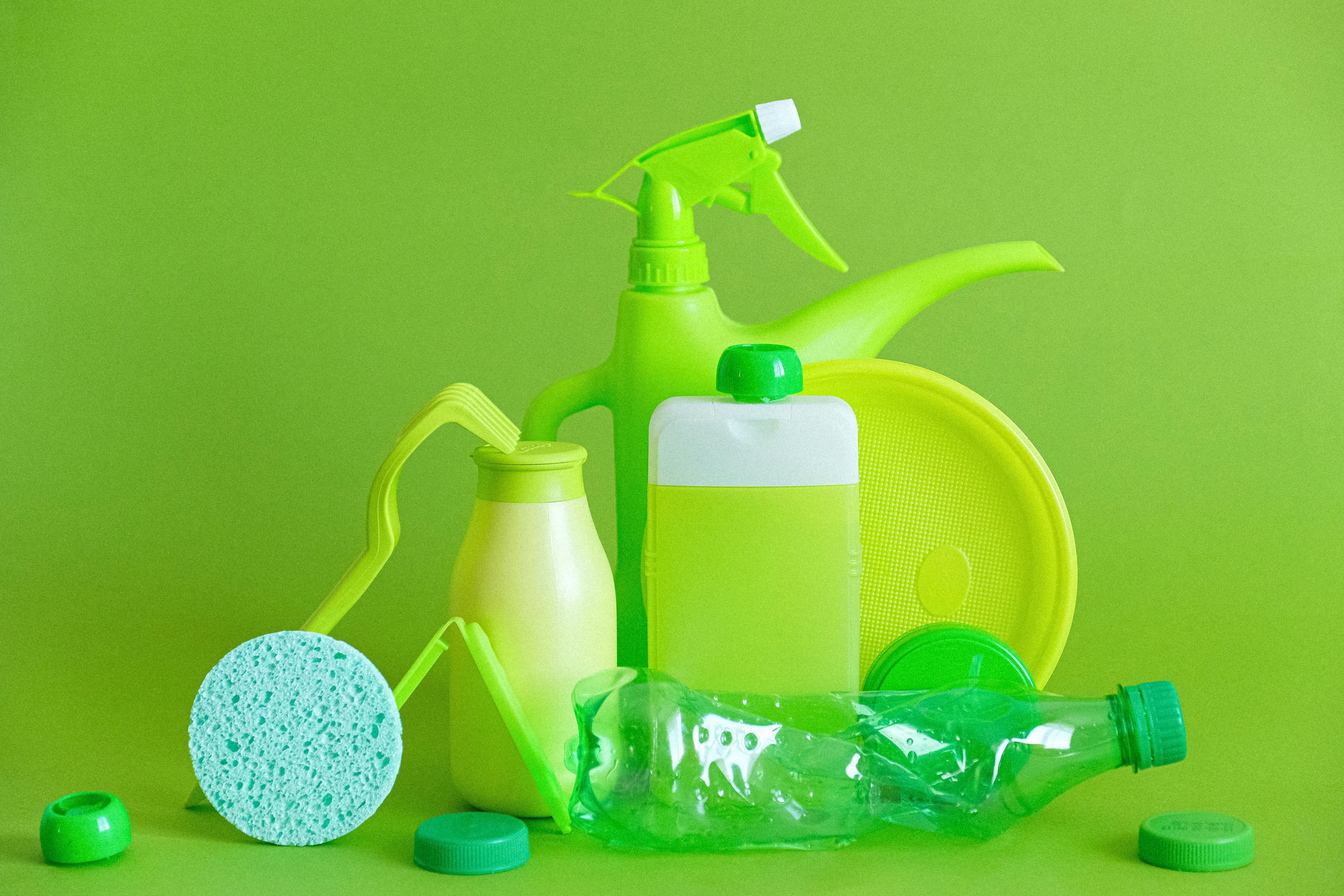
How to Effectively Find Degrees of Freedom in Statistical Analyses
Understanding how to find degrees of freedom is essential for anyone involved in statistical analyses, whether in academia or industry. Degrees of freedom (DF) play a pivotal role in various statistical tests including t-tests, ANOVA, and chi-square tests. This article will explore the concept of **degrees of freedom**, the relevant **degrees of freedom formulas**, and practical applications that demonstrate their importance in statistical results. By the end, you'll be well-equipped to calculate and interpret degrees of freedom in a variety of statistical situations.
Understanding Degrees of Freedom
Degrees of freedom refer to the number of independent values or quantities that can be assigned to a statistical distribution. It is a **critical component of statistical analysis** that deeply influences the reliability of hypothesis testing and the accuracy of statistical estimations. In simplest terms, degrees of freedom are often thought of as an indication of the amount of information available in the data set. This concept is notably significant when comparing variability in sample data with the variability expected under a specific statistical model.
Defining Degrees of Freedom
Defining degrees of freedom requires an understanding of the context within which statistical testing occurs. The general formula for degrees of freedom in a set is calculated by subtracting the number of constraints or parameters from the number of total observations. For instance, in the context of **sample size and degrees of freedom**, if you are conducting a t-test with a sample size \( n \), the degrees of freedom are computed as \( n-1 \). This adjustment accounts for the fact that one parameter (the sample mean) is constrained by the sample data.
Importance of Degrees of Freedom in Statistical Tests
The importance of degrees of freedom is often highlighted in hypothesis testing, particularly when determining statistical significance. Each statistical test utilizes degrees of freedom in its calculations, and these values affect critical thresholds used for determining the test outcomes. For example, in **degrees of freedom for t-tests**, the calculated t-value must be compared against values from the t-distribution that depend on the identified degrees of freedom. Inadequate comprehension of this concept may lead to incorrect interpretations of results, impacting decision-making processes in practical applications.
Calculating Degrees of Freedom: Key Examples
To effectively compute degrees of freedom, it helps to consider common statistical tests. For instance, in a one-sample t-test, the formula for degrees of freedom is simply \( n-1 \), where \( n \) is the number of observations. However, for ANOVA—a pivotal test used in comparing means between groups—the formula generally becomes \( n - k \), where \( n \) is the total number of observations and \( k \) represents the number of groups. A comprehensive understanding of these calculations, including the various **degrees of freedom calculations examples**, demonstrates how they apply in real-world scenarios. Indeed, knowing how to navigate this can significantly enhance the precision of normal evaluations.
Degrees of Freedom in Hypothesis Testing
The utilization of degrees of freedom is an integral part of hypothesis testing in statistics. By understanding how degrees of freedom work within various testing frameworks—like the **degrees of freedom in hypothesis testing**—analysts can interpret test results with accuracy. Hypothesis tests often hinge on the relationship between sample size, **degrees of freedom**, and the variability within a dataset.
Degrees of Freedom for t-Tests
In t-tests, calculating degrees of freedom is vital since they determine how the t-distribution behaves under varying conditions. For independent and paired t-tests, the appropriate formulas vary slightly. For independent t-tests, the formula is \( (n_1 - 1) + (n_2 - 1) \), while for paired t-tests, it's simply \( n - 1 \) where \( n \) is the number of pairs. This differentiation illustrates the nuances in **degrees of freedom in statistical testing** that researchers must grasp to make valid inferences from their data.
Applying Degrees of Freedom in ANOVA
ANOVA, or Analysis of Variance, is commonly employed to evaluate variance across different groups. The **degrees of freedom in ANOVA** can be dissected into two key components: the between-groups and within-groups contributions. The between-groups degrees of freedom equals \( k - 1 \), where \( k \) is the number of groups. Conversely, the within-groups degrees of freedom is calculated as \( n - k \). Recognizing these distinctions can significantly affect the analysis, ultimately highlighting the necessity of rigorous calculations of degrees of freedom in any ANOVA-based study.
Significance of Degrees of Freedom in Regression Analysis
An equally crucial area where degrees of freedom come into play is regression analysis. **Degrees of freedom in regression** are important as they help delineate how much explanatory power a model can claim relative to the variability in data. The total degrees of freedom in regression is based on the sample size reduced by the number of predictor variables being used. This situation elucidates how adding predictors can affect the degrees of freedom, potentially leading to overfitting if not properly managed.
Advanced Applications of Degrees of Freedom
Beyond basic calculations, **degrees of freedom have wide-ranging applications** in fields such as quality control and survey analysis. Understanding degrees of freedom in more complex scenarios is essential for performing rigorous statistical tests accurately.
Degrees of Freedom in Quality Control
In quality control, **degrees of freedom** facilitate the implementation of statistical process control (SPC) techniques to monitor production processes. For instance, control charts use degrees of freedom to compute limits that may signify whether production within a process is statistically stable or requires intervention. Navigating these controls requires critical thinking about how changing degrees of freedom can affect an organization’s ability to maintain quality standards.
Practical Applications in Survey Analysis
The significance extends to survey analysis as well, where sampling distribution adjustments are fundamental. Understanding how to calculate **degrees of freedom** in context becomes important when analyzing competitive surveys. Resources must be allocated effectively, thus emphasizing the interplay between different forms of analysis and how degrees of freedom may lead to more efficient design and interpretation processes.
Implications of Degrees of Freedom in Advanced Statistical Modeling
Moreover, in more complex statistical methodologies, **degrees of freedom calculations** inform model selection. When comparing multiple statistical models, recognizing the degrees of freedom allows experts to evaluate goodness-of-fit effectively while adjusting for model complexity. This deeper exploration of **types of degrees of freedom** is critical in fields ranging from econometrics to bioinformatics, ensuring that analyses yield reliable results.
Key Takeaways
- Understanding how to find degrees of freedom is essential for almost all statistical analyses.
- Correct calculations of degrees of freedom impact the validity of hypothesis tests and regression models.
- Degrees of freedom are pivotal in various statistical tests, including t-tests, ANOVA, and regression analysis.
- Advanced applications span quality control, survey analysis, and mathematical modeling in several fields.
FAQ
1. How do you calculate degrees of freedom in a chi-square test?
The formula for determining **degrees of freedom for chi-square tests** is calculated by the product of the number of rows minus one and the number of columns minus one: \( (r - 1)(c - 1) \). This formula ensures that you accurately assess the independence of categorical variables within a contingency table.
2. Why are degrees of freedom important in regression analysis?
In regression analysis, degrees of freedom are crucial because they determine how well the model fits the data while penalizing for the number of predictors included. The degrees of freedom can signify overfitting if the number of predictors is too high relative to the sample size, which can lead to less reliable estimates of model performance.
3. What is the relationship between sample size and degrees of freedom?
The relationship between sample size and degrees of freedom is that degrees of freedom are usually calculated based on the sample size. An increase in sample size generally leads to an increase in degrees of freedom, which can enhance the robustness of statistical estimates. However, this needs to be balanced with the complexity of the model being tested.
4. Can you provide examples of degrees of freedom adjustments in statistical analyses?
One common **example of degrees of freedom adjustments** is in estimating variance from sample means, often reduced by one to account for a constraint. For ANOVA, similar adjustments apply when assessing multiple means/variances simultaneously, emphasizing their role in accurately defining variability and statistical significance.
5. How does degrees of freedom affect accuracy in statistical results?
Degrees of freedom have a direct impact on the accuracy of statistical results. Specifically, they influence confidence intervals and hypothesis testing outcomes. A well-calculated degrees of freedom can enhance the credibility of parameter estimates, making conclusions drawn from statistical tests more reliable.