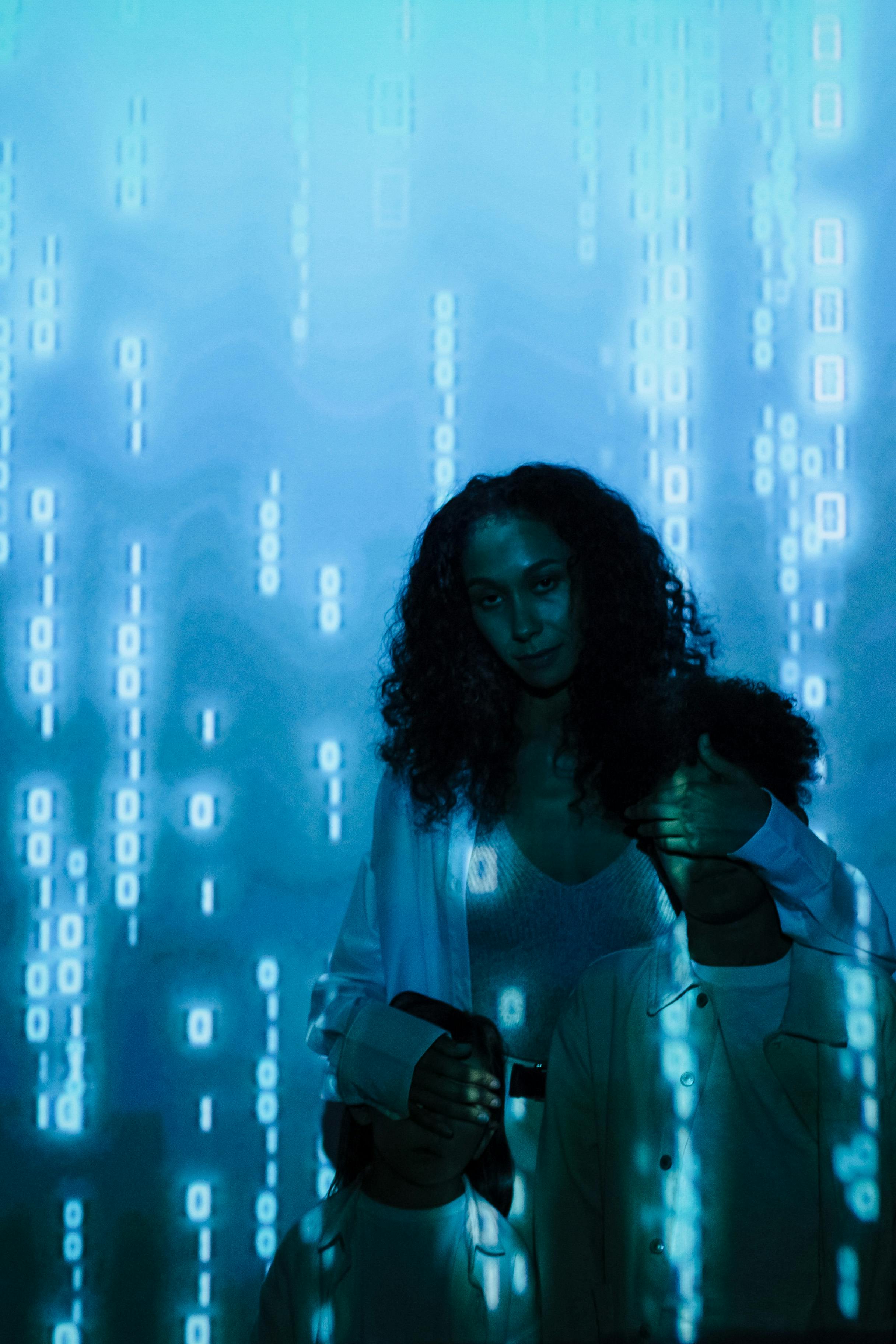
How to Calculate the Interquartile Range: An Updated Guide for 2025
Understanding the concept of the interquartile range (IQR) is crucial for anyone delving into data analysis. Knowing how to calculate the interquartile range provides a foundation for analyzing data variability and interpreting values effectively. This guide will equip you with the necessary knowledge to confidently find the interquartile range, explore its significance, and apply it in various statistical contexts.
Understanding Interquartile Range
The interquartile range definition refers to the difference between the upper quartile (Q3) and the lower quartile (Q1) in a dataset. This statistical measure provides insight into the spread of the middle 50% of data points, helping to identify the central tendency and variability within a dataset. The interquartile range formula is simple: IQR = Q3 - Q1. By focusing on the interquartile range, analysts can minimize the influence of outliers and extreme values that may skew data interpretations.
Quartiles in Data Analysis
Quartiles divide a data set into four equal parts, yielding three critical values: the first quartile (Q1), the second quartile (Q2 or median), and the third quartile (Q3). To better understand finding the median and quartiles, consider arranging your data from smallest to largest, then identify Q1, Q2, and Q3. The quartiles represent values at which certain percentages of the data fall. For instance, Q1 marks the 25th percentile, while Q3 marks the 75th. This division illustrates how data is distributed across the range, giving insights into data trends and behavior.
Steps to Find Interquartile Range
Here are the steps to find the interquartile range effectively: 1. **Arrange the Data**: Sort your dataset in ascending order. 2. **Calculate Quartiles**: Find Q1 and Q3 using the formulas or statistical tools. 3. **Apply the IQR Formula**: Substitute Q1 and Q3 into the IQR formula (IQR = Q3 - Q1). 4. **Interpret the Results**: Consider how the interquartile range reflects data concentration and outliers. This step is crucial for conducting comprehensive statistical analysis.
Interpreting Interquartile Range
Recognizing the significance of interquartile range in data analysis cannot be overstated. It expresses how data varies and provides insights into the overall distribution, which is particularly vital for assessing normalcy in datasets. Unlike range, which can be heavily influenced by outliers, the IQR provides a more robust understanding of data spread by focusing solely on the middle segments.
Interquartile Range Applications
The use of interquartile range extends across multiple fields, including finance, biology, and social sciences. For example, in finance, analysts might use the IQR to assess stock price volatility, while in biology, researchers might analyze test scores using the IQR to determine learning patterns among students. Understanding IQR applications leads to better-informed decisions and insights into data-driven conclusions.
Interquartile Range and Outliers
Identifying outliers is critical in statistical analysis, and the interquartile range plays a significant role here. Outliers are commonly defined as any observations that lie beyond the lower and upper fences, which can be calculated using the formula Lower Fence = Q1 - 1.5 * IQR and Upper Fence = Q3 + 1.5 * IQR. Any data point falling below the lower fence or above the upper fence is considered an outlier. This relationship helps analysts maintain data integrity by excluding misleading observations in their analyses.
Visualizing the Interquartile Range
Effectively visualizing IQR can greatly enhance comprehension and presentation of data. Box plots (or box and whisker plots) are commonly used to display the interquartile range graphically. These plots show the quartiles, median, and potential outliers, providing a clear view of how data points are dispersed around the median. It is an excellent tool for communicating complex statistical concepts to audiences with varied backgrounds.
Box Plot Interquartile Range Example
To better illustrate how to visualize the interquartile range, let’s consider an example. Suppose we have the following dataset: [12, 14, 16, 20, 22, 23, 27, 30, 34]. First, we organize the data and determine the quartiles. For this dataset: - Q1 (lower quartile) is 20. - Q3 (upper quartile) is 27. Thus, the IQR would be IQR = 27 - 20 = 7. A box plot would show a box from Q1 to Q3, with a line at the median. This visualization allows for immediate understanding of data distribution and outlier detection.
Calculating Quartiles in Different Scenarios
Different methods exist for calculating quartiles, particularly when dealing with larger datasets or those with repeated values. For example, the nearest ranks method involves positioning the rank of data points based on their order. Conversely, linear interpolation is useful when calculating quartiles among continuous data, providing a more precise measure. Comprehending these various techniques enhances the ability to calculate quartiles accurately.
Key Takeaways
- The IQR measures the middle 50% of data variability and protects against outliers' influence.
- Learning how to calculate the interquartile range involves understanding quartiles and employing a straightforward formula.
- Utilizing box plots can significantly enhance data interpretation through effective visualization.
- Applying the IQR in various fields provides essential insights for making data-driven decisions.
- Interpreting outliers through the IQR informs about data integrity and stability.
FAQ
1. What is the purpose of finding the interquartile range?
The primary purpose of finding the interquartile range (IQR) is to assess data variability. It offers insights into data dispersion and helps in identifying outliers, thereby allowing analysts to make more accurate interpretations of the dataset.
2. How can the interquartile range be useful in data analysis?
The IQR is vital in data analysis as it measures the spread of the central value, fixes issues presented by outliers, and provides a clearer understanding of data distributions, leading to more informed decisions in research and statistical evaluations.
3. How do you calculate quartiles besides the IQR?
Calculating quartiles typically involves sorting the dataset, finding the median, and using the median to divide the data into two halves to calculate Q1 and Q3. Various methods exist, including the nearest ranks and linear interpolation approach.
4. Can I use the interquartile range for any type of data?
While the IQR can be applied to various datasets, it is particularly effective for continuous and ordinal data witnessed in areas like social sciences and natural sciences. However, it may not be suitable for nominal data where median calculations do not make sense.
5. How can I effectively visualize the interquartile range?
Box plots are widely recognized for visualizing the interquartile range. These plots visually represent the quartiles and median while identifying potential outliers, providing a clear depiction of the spread and variations within the data.
6. What are common mistakes when calculating the interquartile range?
Common errors include calculating quartiles incorrectly by misplacing values, failing to sort data, or misunderstanding how to apply the IQR formula. These mistakes can lead to inaccurate interpretations of data and misleading conclusions.
7. How does the IQR relate to data skewness?
The IQR can help identify data skewness by comparing values within the IQR to the overall data distribution. A smaller IQR in relation to the entire range might indicate skewed data, while a larger IQR suggests a more uniform distribution.