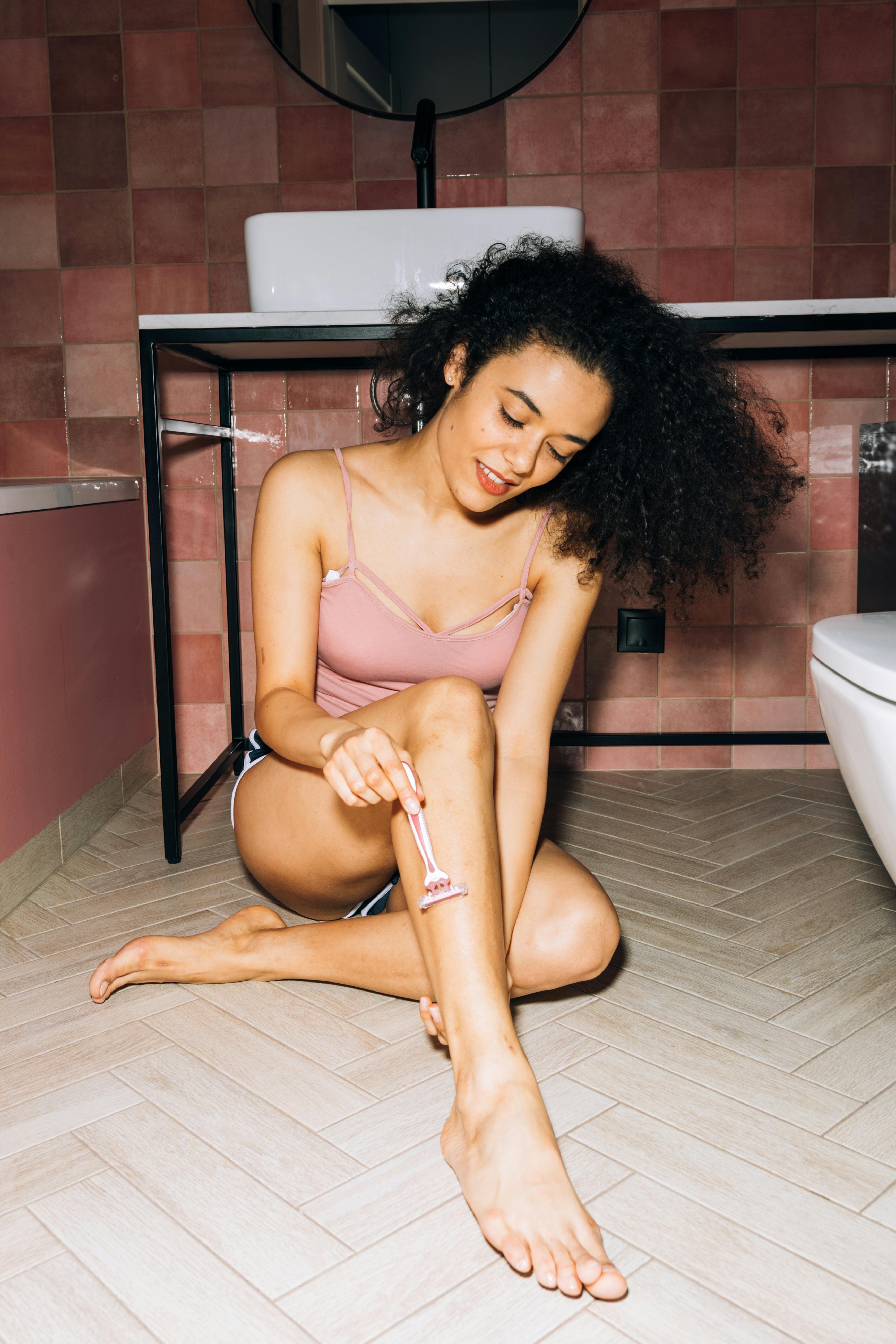
Essential Guide to Understanding the P-Value in 2025
The p-value is a cornerstone concept in statistics, particularly within the fields of hypothesis testing and statistical significance. By providing a measure of how compatible your data is with the null hypothesis, it plays a vital role in various research methodologies, including experimental design and observational studies. Whether you’re conducting clinical trials or engaging in data analysis, grasping the nuances of the p-value is fundamental for making informed decisions based on statistical evidence.
This article will delve into effective ways to find and interpret the p-value in 2025. By exploring various statistical tests such as the t-test, chi-square test, and ANOVA, we will also discuss concepts like the significance level, critical value, and the impact of sample size on p-values. Furthermore, we will shed light on common misconceptions surrounding p-values and the importance of statistical power in research.
Ultimately, understanding the p-value is crucial for anyone involved in quantitative research, as it empowers effective communication of results and enhances the reliability of conclusions drawn from statistical analyses. Get ready to unlock the secrets of the p-value!
How to Calculate the P-Value in Hypothesis Testing
Calculating the p-value is essential in hypothesis testing as it helps determine whether to reject or fail to reject the null hypothesis. This process typically involves the following steps:
Step-by-Step Process for p-value Calculation
To calculate the p-value, begin by formulating your null and alternative hypotheses. Next, choose the appropriate statistical test based on your data's characteristics. Depending on the type of data and hypotheses, you may select from tests like t-tests, chi-square tests, or ANOVA. After calculating the test statistic corresponding to your chosen test, you'll find the p-value using statistical tables or software solutions like p-value calculators.
Choosing the Right Statistical Test
The choice of a statistical test is crucial when determining the p-value. For instance, a t-test is appropriate for comparing means, whereas a chi-square test is used for categorical data. Importantly, understanding the nature of your data will guide you toward the most effective choice. In 2025, predictive modeling and machine learning techniques can also simplify this process by recommending appropriate statistical methods based on input data.
Understanding Statistical Significance Levels
The significance level, often denoted as alpha (α), is a threshold that helps make sense of the p-value in hypothesis testing. Commonly set at 0.05, it indicates a 5% chance of committing a Type I error, where you incorrectly reject a true null hypothesis. Understanding this relationship is vital for proper interpretation of results, as it allows researchers to balance the risks associated with false positives and negatives.
Importance of Sample Size in P-Value Estimation
Sample size plays a pivotal role in influencing p-values. Larger sample sizes generally lead to smaller p-values as they provide more reliable estimates of population parameters. In practice, researchers should consider the implications of sample size when designing studies. Statistical power, which reflects the likelihood of correctly rejecting a false null hypothesis, is also affected by sample size, together emphasizing the need for adequate planning in research methodologies.
Examples of P-Value Calculations
Let’s consider a simple example. In a clinical trial comparing two treatments, you might start with a null hypothesis stating that both treatments have the same effect. After conducting a t-test, if you find a p-value of 0.03, this indicates strong evidence against the null hypothesis, particularly if your significance level is set at 0.05. Conversely, a p-value of 0.08 would suggest insufficient evidence to reject the null hypothesis.
Interpreting P-Values Accurately
With calculations complete, the next step is accurate p-value interpretation. Misinterpretation can lead to flawed conclusions in research. Understanding what a p-value does and does not signify is critical for valid statistical inference.
Common Misconceptions About P-Values
One widespread misconception is that a p-value indicates the probability that the null hypothesis is true. Instead, it tells us the probability of obtaining results as extreme as those observed, given that the null hypothesis is true. Moreover, there's a nuance regarding the effect size and practical significance. A small p-value does not necessarily indicate a strong effect; researchers need to consider effect size in their analyses to gauge practical relevance.
Bayesian vs. Frequentist Interpretations of P-Values
In recent years, there has been increasing interest in Bayesian statistics as an alternative to traditional frequentist methods. While the frequentist approach relies heavily on p-values, Bayesian statistics allows for more nuanced interpretations, incorporating prior evidence and calculating probabilities of hypotheses directly. Understanding these differences can enhance researchers' engagement with these statistical frameworks, ultimately leading to better data-driven decision making.
Reporting P-Values in Research
When reporting p-values in scientific research, clarity is paramount. Researchers should provide context regarding the tests conducted, assumptions made, and how the p-value relates to the research questions. Accurate reporting not only improves transparency but also enhances reproducibility of results in the scientific community. Guidelines around proper reporting of p-values continue to evolve, encouraging researchers to uphold ethical considerations in their scientific endeavors.
The Role of P-Values in Clinical Trials
In clinical trials, p-values are utilized to determine the efficacy of new treatments. By establishing a null hypothesis that posits no difference between treatment groups, clinical researchers analyze data to calculate p-values, guiding decisions around treatment approvals and clinical guidelines. Understanding how p-values contribute to research conclusions is essential for both researchers and practitioners in making informed decisions about patient care and treatment methodologies.
Practical Applications of P-Values in Data Analysis
P-values serve critical roles in various fields, from clinical trials and observational studies to social sciences. By integrating p-values into research methodologies, scientists can enhance the robustness of their findings.
Using P-Values in Regression Analysis
In regression analysis, p-values help assess the significance of predictors in relation to an outcome variable. By evaluating the p-values associated with each predictor, researchers can determine which variables contribute meaningfully to the model's explanatory power. Careful interpretation of these p-values can lead to better insights into the relationships between variables, guiding future research directions.
P-Values and Correlation Coefficients
When exploring relationships between two variables, correlation coefficients provide a measure of association, while p-values determine whether the observed correlation is statistically significant. Understanding both measures contributes to credible data analysis, enabling researchers to draw valid conclusions from their findings.
Alternatives to P-Values for Statistical Testing
Although p-values are widely used, there are alternatives for statistical testing that can complement or replace them. Measures such as confidence intervals, effect sizes, and Bayesian methods offer valuable insights beyond mere p-value thresholds. Employing a combination of these methods offers a more comprehensive assessment of research findings.
Utilizing Technology for P-Value Computation
The advancement of data analytics technologies and statistical software has simplified the process of p-value computation. Modern tools can perform complex analyses, including meta-analysis and non-parametric tests, enabling researchers to derive p-values swiftly. Utilizing online p-value calculators further enhances accessibility, allowing more extensive applications across various disciplines.
Graphical Representations of P-Values
Graphical representations can enhance the interpretation of p-values, providing insight into the distribution of data and the significance of results. Visualization techniques, such as box plots and histograms, can display p-values contextually, facilitating comprehension of the data’s statistical landscape.
Q&A: Common Questions About P-Value and Its Interpretation
What is a p-value in statistics?
A p-value is a measure that helps you determine the strength of evidence against the null hypothesis. It quantifies the probability of observing data at least as extreme as the data collected, assuming the null hypothesis is true.
How do I calculate the p-value for my study?
To calculate the p-value, select the appropriate statistical test for your analysis (e.g., t-test, ANOVA). Compute the test statistic, then use statistical tables or software to find the corresponding p-value. Online p-value calculators can also streamline this process.
What are common p-value thresholds for significance?
Common p-value thresholds for significance are 0.05, 0.01, and 0.001. A lower threshold indicates stricter criteria for rejecting the null hypothesis and suggests increased confidence in the findings.
How does sample size affect the p-value?
Sample size significantly influences p-values; larger samples generally yield smaller p-values, improving the accuracy of the estimates of the population parameters. Adequate sample size is crucial for enhancing statistical power in hypothesis testing.
Are there limits to using p-values in research?
Yes, p-values have limitations, including misunderstanding by researchers and misuse in interpreting statistical significance. It is vital to complement p-values with effect sizes and confidence intervals for a more comprehensive understanding of study results.
Conclusion: Mastering the P-Value for Better Research Outcomes
In 2025, understanding and accurately interpreting the p-value remains essential for researchers across disciplines. By recognizing its role in hypothesis testing and its implications for significance levels and statistical power, you can enhance the quality and reliability of your research outcomes. Employing alternative methods and being aware of common misconceptions enriches your toolkit for data-driven decision making.
As you continue to explore statistical methodologies, remember that p-values are just one piece of the puzzle in comprehensive data analysis. Adopting a holistic approach, combining p-values with effect sizes and confidence intervals, will elevate your research practices and lead to more informed scientific discourse.