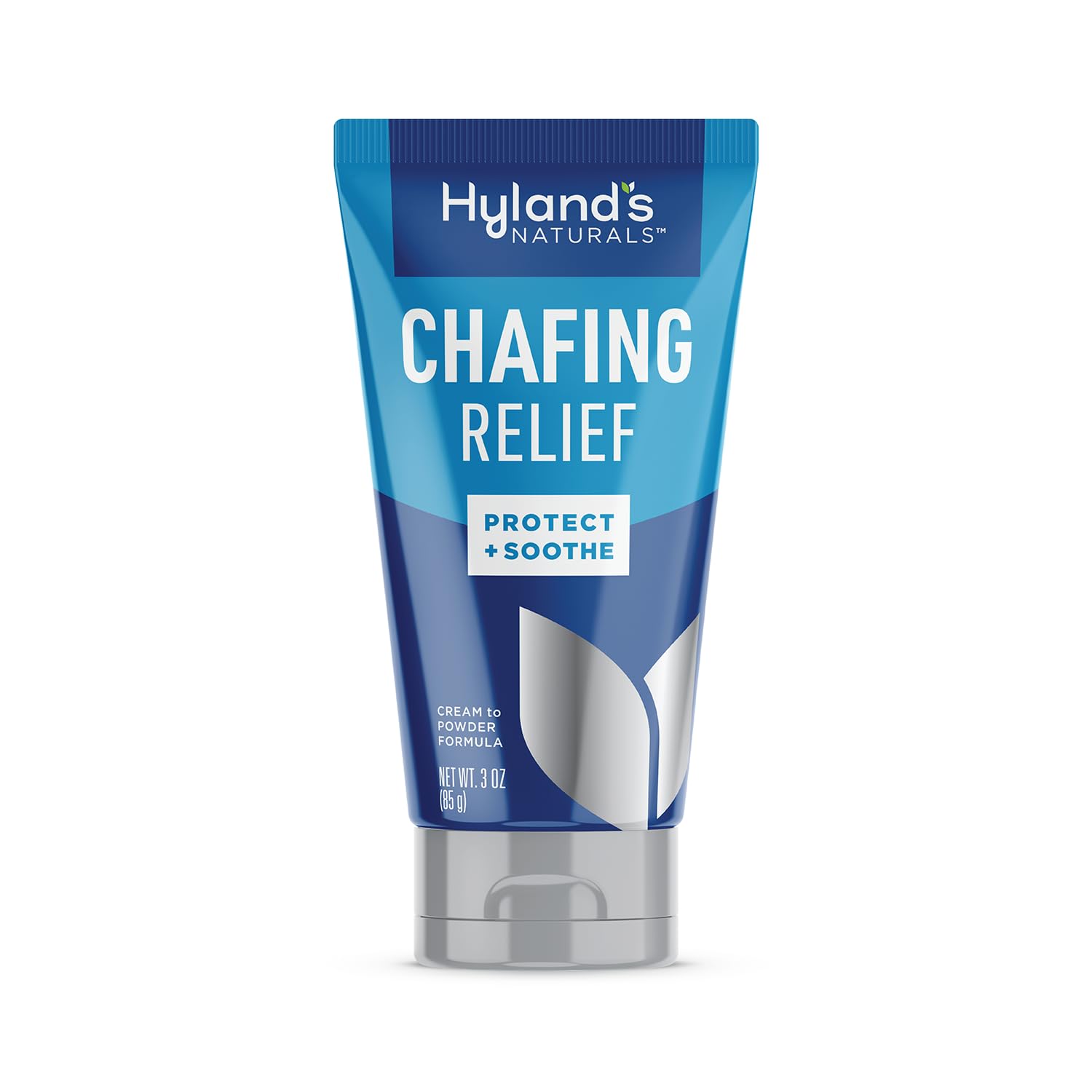
How to Find the Interquartile Range: Essential Steps for Accurate Data Analysis in 2025
The interquartile range (IQR) is a crucial statistical measure that helps identify the spread and variability within a data set. Understanding **how to find the interquartile range** effectively can enhance your data analysis, allowing for better insights and decision-making. In 2025, as data becomes increasingly vital across various fields, mastering the techniques for **calculating the IQR** will be essential for researchers, analysts, and anyone working with data. This article explains the **steps to calculate IQR**, provides examples, and highlights its significance in data analysis.
Understanding Quartiles and Their Importance
Quartiles divide a data set into four equal parts, each representing a quarter of the values. The **lower quartile (Q1)** is the median of the lower half of the data, the **upper quartile (Q3)** is the median of the upper half, and the **median (Q2)** is the overall middle value. Knowing these quartiles is fundamental when it comes to **finding IQR in statistics**. In science and research, understanding quartiles allows for effective **data spread analysis**, which is integral to accurate **data interpretation methods** and identifying clustering patterns or divisions in data distribution.
The Concept of Interquartile Range Explained
The **interquartile range** is calculated as follows: \( IQR = Q3 - Q1 \). This formula provides an essential measure of variability, as it captures the range of the middle 50% of data points, thus reducing the influence of extreme values. **Determining IQR values** is important not just for summarizing data but for understanding statistical significance and managing data variability. By focusing on the IQR, population averages are less skewed by outliers, producing a clearer picture of the data set's central tendency. Accordingly, knowing the **IQR in box plots** ⭐ helps visually represent data relationships and distribution, making it easier to identify outliers and potential anomalies.
Practical Examples of Calculating IQR
Let's consider a practical example to see how the IQR is calculated. Imagine we have the following data set representing exam scores: 55, 70, 50, 80, 90, 85, 60, 75. To find the IQR, first sort the data: 50, 55, 60, 70, 75, 80, 85, 90. The median (Q2) here is 72.5, while: - Lower quartile (Q1) is the median of 50, 55, 60, 70: (55 + 60) / 2 = 57.5 - Upper quartile (Q3) is the median of 75, 80, 85, 90: (80 + 85) / 2 = 82.5 The **IQR calculation** is then \( IQR = 82.5 - 57.5 = 25 \). This **IQR** helps characterize the exam scores' spread, informing educators about the overall performance distribution. More importantly, understanding quartiles and employing the IQR effectively can assist in better-performance evaluations and data-driven decisions.
Steps to Calculate IQR
Calculating the IQR involves a systematic approach. These step-by-step instructions simplify the process of **finding IQR in data sets**, ensuring accurate results for insightful analysis.
Step 1: Organize Your Data
The first step to finding the IQR is to sort your data set in ascending order. This order is essential for accurate **quartile calculations**. For example, given the data points: 12, 8, 10, 15, 14, arrange them as 8, 10, 12, 14, 15. Organization is crucial for effective analysis and enhances data integrity during your processing. After organizing, you can easily identify the relevant quartiles needed for IQR calculation.
Step 2: Identify the Quartiles
Next, determine the lower (Q1) and upper (Q3) quartiles. For the arranged data (8, 10, 12, 14, 15), the steps might vary slightly based on whether the data set has an odd or even number of items. You can calculate Q1 and Q3 using medians for their respective halves of the data. Calculating quartiles allows you to gain insights into your data spread and skewness, which is beneficial in understanding data distribution impacts.
Step 3: Calculate the IQR
Finally, apply the IQR formula \( IQR = Q3 - Q1 \). This step summarizes the variability in your data effectively. An example can demonstrate this: If Q1 is 10 and Q3 is 14, then \( IQR = 14 - 10 = 4 \). Knowing the IQR helps in identifying potential outliers within the data set, enabling data validation techniques and reinforcing the act of **using IQR for outliers**. The significance of the IQR becomes evident through these calculations as it serves as a direct measure of data spread, thereby guiding your decision-making data clarity.
Visualizing IQR for Enhanced Analysis
Visualization plays a crucial role in understanding complex data. By incorporating **IQR in visual representation such as box plots**, statisticians and researchers can grasp distributions profoundly and efficiently. Box plots visually summarize data sets with important summary statistics, typically including the median, quartiles, and potential outliers. These graphics promote effective data analysis, allowing for clearer comparisons and results interpretation.
Creating Box Plots: A Hands-On Example
To visualize the previous exam score data set (50, 55, 60, 70, 75, 80, 85, 90) as a box plot, start by marking the quartiles along a number line. Q1 is at 57.5, Q2 at 72.5, and Q3 at 82.5. Draw a box extending from Q1 to Q3 with a line inside marking the median. Any scores outside Q1 - 1.5*IQR or Q3 + 1.5*IQR can be considered as potential outliers. This graphic representation not only indicates data variability but significantly illustrates crucial data quality aspects, reaffirming your insights from effective statistical analysis and interpretation.
Using IQR for Data Interpretation
Finally, understanding performance and variability with the IQR can be applied in many fields, such as finance, education, and health. By assessing data integrity through the lens of the IQR, organizations can determine compliance or efficiency in their systems. When applied across diverse research studies, the **significance of IQR** extends into broader conclusions about trends and behaviors, contributing to better decision-making strategies.
Key Takeaways
- The **IQR formula** provides a comprehensive measure of variability in data sets.
- Steps to calculate IQR include organizing data, identifying quartiles, and applying the IQR formula.
- Visualizing IQR, especially through box plots, enhances understanding of data spread and outliers.
- Utilizing IQR effectively has applications in educational assessments, finance, and research studies.
- Grasping quartile significance leads to better data analysis and informed decision-making.
FAQ
1. How is the IQR used in identifying outliers?
The IQR plays a significant role in identifying outliers by establishing limits beyond which data points can be considered atypical or extreme. Specifically, data points less than \( Q1 - 1.5 \times IQR \) or greater than \( Q3 + 1.5 \times IQR \) are classified as outliers. This method is vital for effective data validation techniques and ensures cleaner, more reliable datasets.
2. Can IQR help in understanding data variability?
Yes, the IQR efficiently summarizes data variability by focusing on the central 50% of the data, thereby minimizing the effects of extreme values. This measure is critical for assessing spread within various data sets, leading to improved **data spread analysis** and ultimately better **statistical reporting**.
3. How does one interpret IQR in research studies?
In research, the **IQR** is interpreted as an indicator of variability and spread, providing insights into consistency and homogeneity within the data. A smaller IQR suggests that the data points are closely clustered around the median, while a larger IQR indicates more diversity. Understanding the IQR aids in reinforcing the significance of reported findings.
4. What is the significance of quartiles in box plots?
Quartiles are integral to box plots as they visually represent data spread. Box plots illustrate the range of the data through the quartiles, help identify central tendencies, and highlight any potential outliers, thus enhancing the capability for effective data analysis. They provide a quick yet comprehensive overview of data distributions and variability.
5. How do I apply IQR in statistical analysis?
To apply the IQR in statistical analysis, first calculate the quartiles then compute the IQR. This information can be leveraged when examining data distributions, ensuring the precision of assessments, and conducting comparative analysis. Proper usage of IQR assists researchers and analysts in extracting reliable insights from their data.
6. Is IQR applicable to non-normally distributed data?
Absolutely. The IQR is a robust statistic useful for all types of data distributions, especially when the data is skewed or contains outliers. This characteristic makes the IQR an essential tool in descriptive statistics, ensuring the reliability of analyses across various fields.
7. What tools can be used to calculate IQR in large data sets?
There are multiple software tools available for calculating the IQR in large datasets, such as Python libraries (like Pandas or NumPy), R programming, and several statistical analysis software programs like SPSS or SAS. These tools significantly streamline both the computational effort and the **steps to calculate IQR**, making them highly efficient for data professionals and researchers.