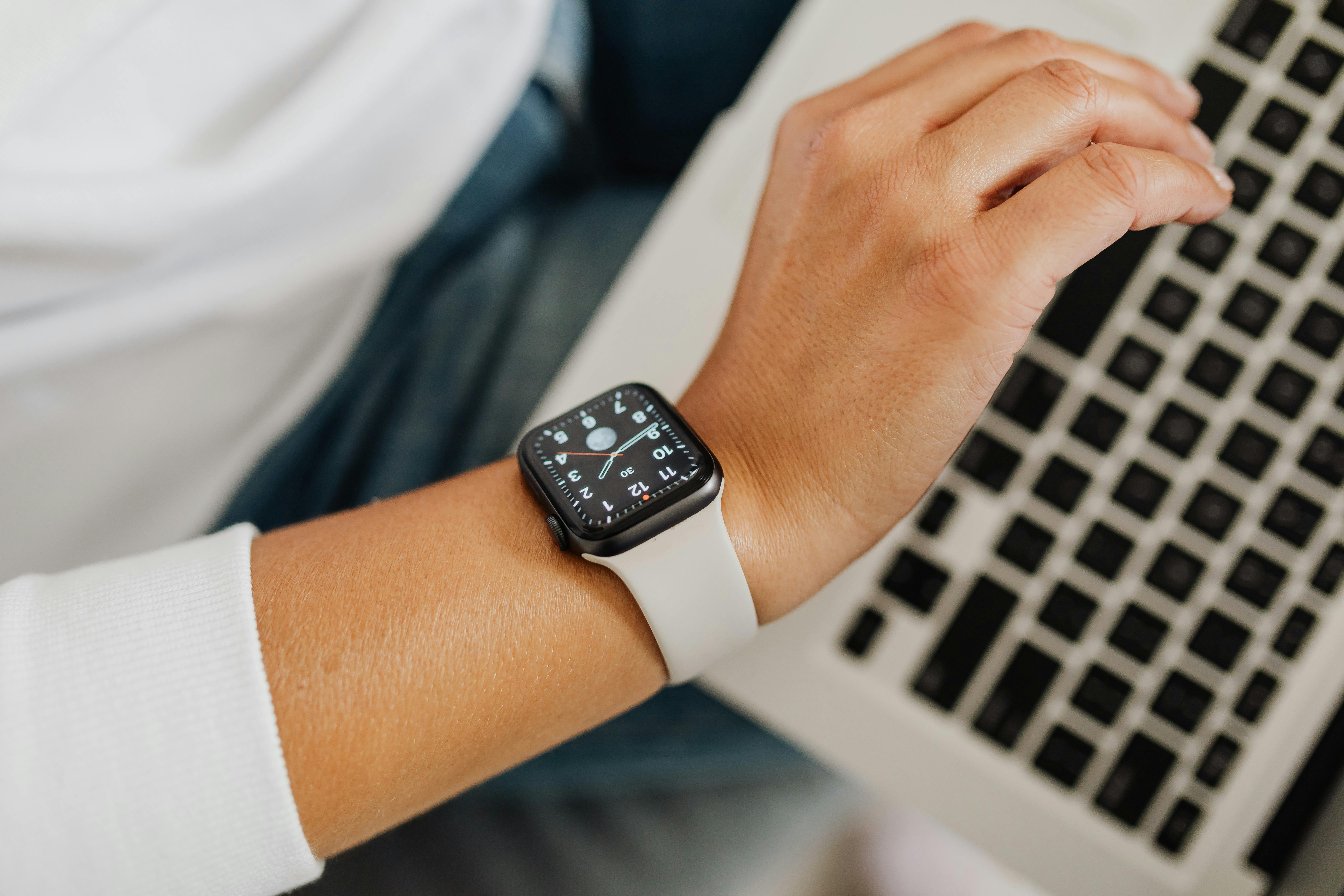
Smart Guide to Effectively Calculating Margin of Error in 2025
Understanding the Margin of Error and Its Importance
Margin of error is a crucial factor in statistical analysis, particularly in surveys and polling data. It quantifies the uncertainty in survey results and helps in understanding how close the sample result is to the true population parameter. As we delve into 2025, the significance of accurately calculating margin of error remains paramount, especially with the increasing complexity of statistical measures and data interpretation. This guide aims to equip you with effective methods to calculate margin of error, explore its foundations, practical applications, and the impact on decision-making.
As a key element in research methodology, the margin of error defines the range of potential deviation in sample results from the actual values in the target population. It enhances the reliability of data through proper statistical modeling and evaluation techniques. In this article, we will discuss the margin of error formula, factors influencing its calculation, and provide practical examples to solidify your understanding.
Key takeaways from this guide include:
- An overview of the margin of error and its components.
- Step-by-step instructions on using the margin of error formula.
- Common mistakes to avoid during calculations.
- Insights into the relationship between sample size, confidence level, and margin of error.
Visual aids and practical examples will enhance your comprehension, making it easier to grasp these concepts effectively. Let's embark on our journey through the margin of error calculations.
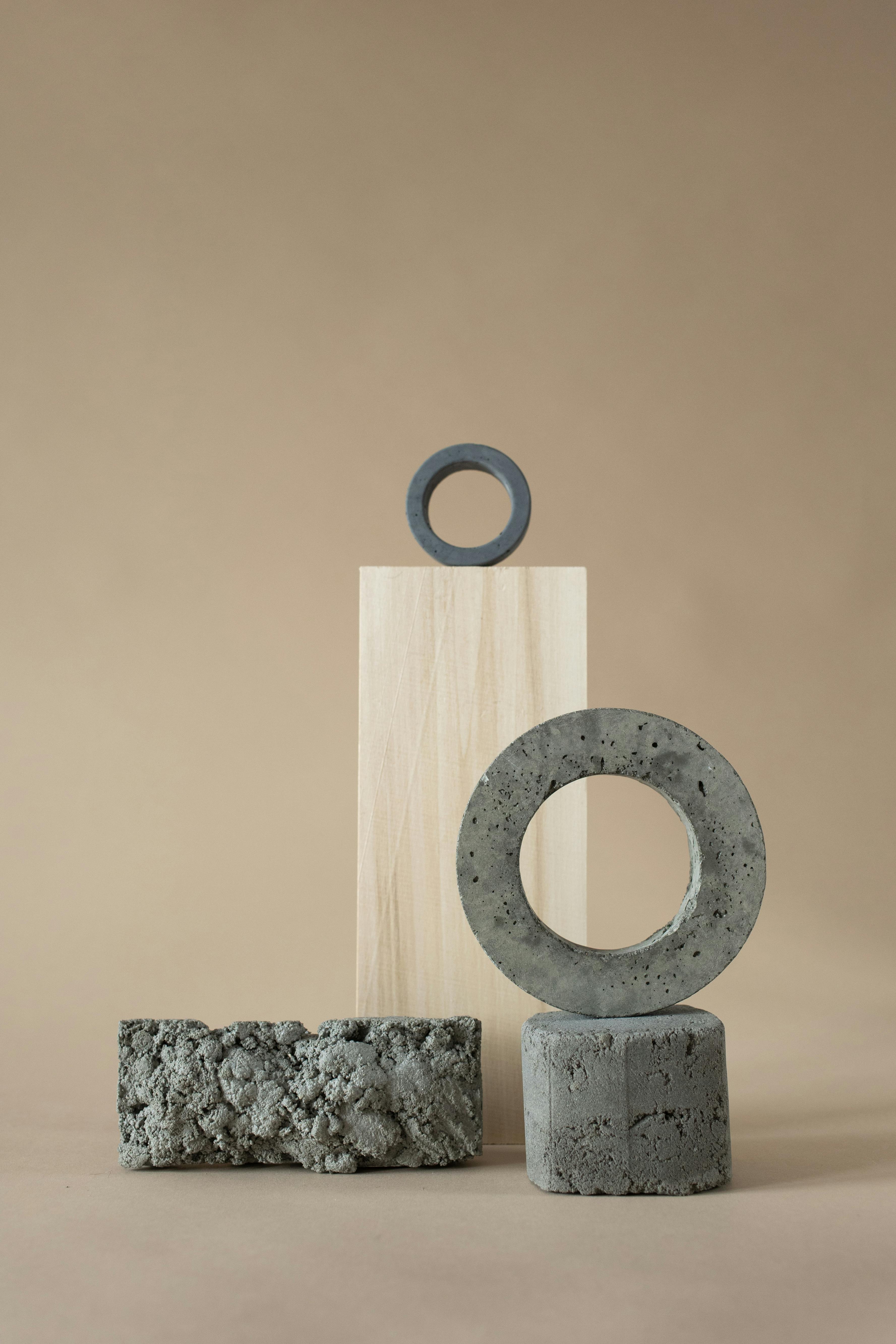
Defining Margin of Error and Its Components
Building on our introduction to margin of error, let's clarify its definition and components. The error margin definition indicates the extent to which survey results might differ from the true population values due to sampling variability. Statistically, it can be expressed as a percentage added to and subtracted from the sample statistic to form a confidence interval.
Typically, margin of error is influenced by:
- Sample Size: Larger samples tend to provide more reliable estimates, resulting in a smaller margin of error.
- Confidence Level: The degree of certainty in the estimation reflects the confidence level chosen (commonly 90%, 95%, or 99%).
- Standard Deviation: This measures the variation within a set of values; higher variability can increase the margin of error.
In essence, the margin of error represents the range of expected variability in survey results, providing a quantitative means to assess accuracy. As we delve deeper, we'll explore how to calculate the margin of error using the appropriate formula and what factors come into play during this process.
Calculating the Margin of Error Formula
With a solid understanding of margin of error components, let's dive into the mechanics of calculating this important statistic. The margin of error formula is typically represented as:
Margin of Error (E) = Z * (σ/√n)
Where:
- Z: Z-score corresponding to the confidence level (e.g., 1.96 for 95% confidence level).
- σ: Standard deviation of the population. If unknown, the sample standard deviation can be used.
- n: Sample size.
To illustrate, consider the following example: You are conducting a survey on student satisfaction in a school with a sample size of 100 students. The standard deviation from previous surveys is 1.2. For a 95% confidence level, the Z-score is 1.96. Plugging these values into the formula gives:
E = 1.96 * (1.2/√100) = 0.2352
This result indicates that the sample results may vary by approximately ±0.2352, giving you the confidence interval of your survey data.
Next, we will explore how sample size influences the margin of error and offer practical advice on determining an appropriate sample size for your research.
The Role of Sample Size in Margin of Error
Having established the margin of error formula, it is essential to highlight how sample size plays a pivotal role in determining the margin of error. As a fundamental aspect of statistical analysis, the sample size significantly affects the accuracy of polling results.
Increasing your sample size can lead to more reliable data and a smaller margin of error due to a more accurate representation of the population. Conversely, a smaller sample size can increase the margin of error, leading to less reliable results. It's crucial to find a balance that ensures adequate representation of the target population while maintaining feasible logistical considerations.
For example, if you are surveying a local community with a population of 1,000, and you choose a sample size of 50, your margin of error will be larger than if you selected a sample size of 200. In practice, as the size n increases, the effect of the standard deviation diminishes on the overall margin of error, which leads to findings that are more generalizable to the entire population.
Practical Steps for Determining Sample Size
To effectively determine an appropriate sample size, follow these practical steps:
- Determine the population size and the estimated proportion of the attribute of interest.
- Select the desired confidence level based on how certain you wish to be about your results.
- Use online sample size calculators or statistical tables to determine the required sample size based on the above factors.
By accurately defining your sample size, you can enhance the precision of your survey and ultimately improve your decision-making processes. In the next section, we’ll delve into common calculations involving margin of error in real-world applications.
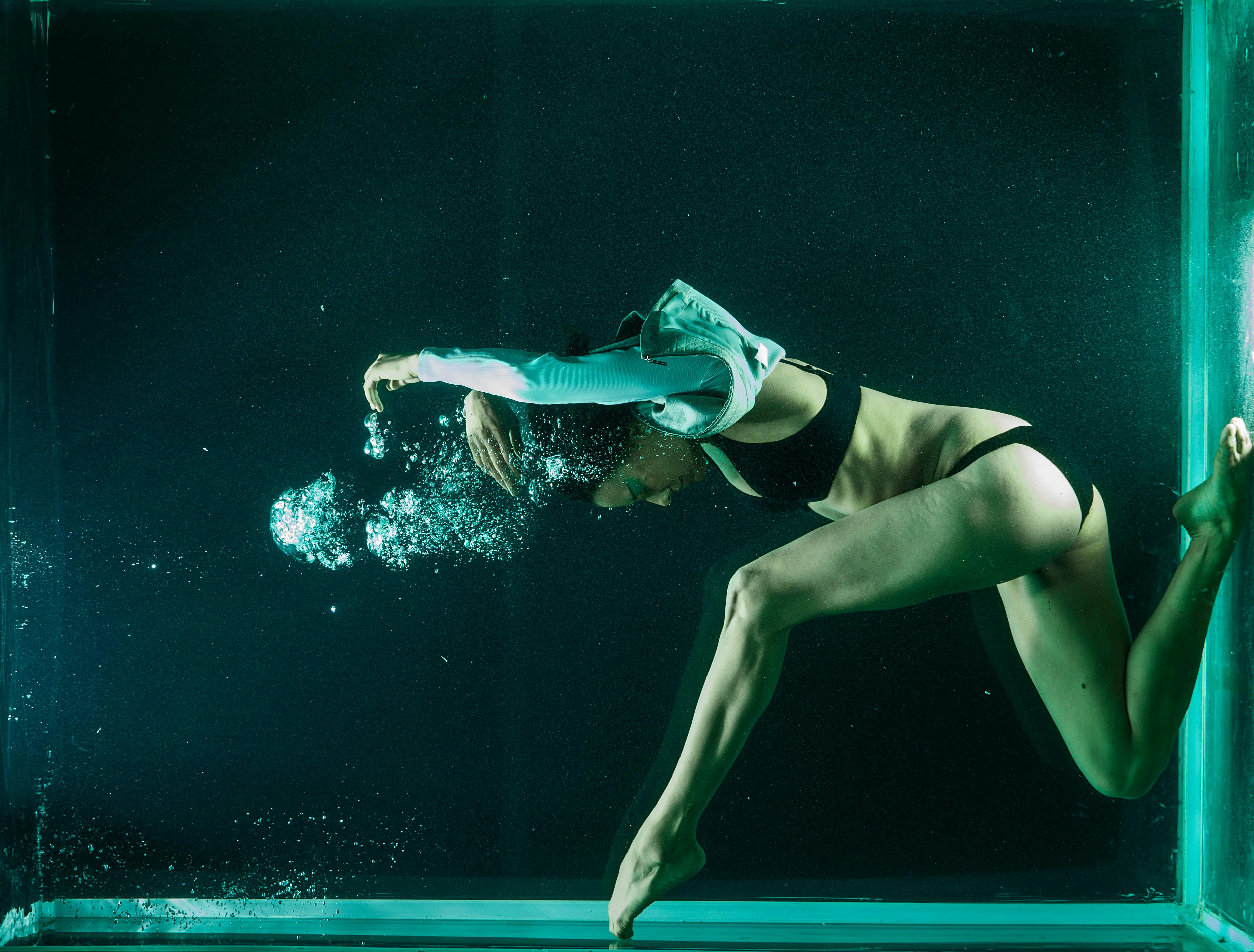
Common Mistakes in Margin of Error Calculations
Being aware of common mistakes in error margin calculations can dramatically enhance the accuracy of your survey results. Many researchers and data analysts inadvertently make oversight errors that can skew their findings. Here are several common pitfalls along with prevention strategies:
1. Ignoring Population Variability: Not accounting for variability can lead to inaccurate estimations. Always analyze prior data to understand variance and adjust your standard deviation accordingly.
2. Miscalculating Sample Size: Underestimating the required sample size can amplify your margin of error. Always return to your goals and the required precision for a reliable gauge.
3. Overlooking the Confidence Level: Selecting an inappropriate confidence level can either overstate or understate your margin of error, impacting the reliability of your research. Consider your audience and application when selecting a confidence level.
By being vigilant and applying best practices during your calculations, you can effectively reduce the risk of error and ensure that your results are reflective of the true population dynamics. In the next section, we will look into statistical significance and its relationship with margin of error.
Interpreting Statistical Significance with Margin of Error
Interpreting statistical significance in relation to margin of error is critical when analyzing survey data. Statistical significance measures whether your survey results are likely to reflect true differences in the population rather than mere random fluctuations.
By comparing your margin of error to the observed effect sizes in your data, you can assess how statistically significant your findings are. A smaller margin of error typically correlates with a higher degree of certainty regarding the survey results' reliability.
When conducting statistical hypothesis testing, consider the confidence interval created using your margin of error. A statistically significant result indicates that the observed differences or relationships in your data are unlikely to have occurred by chance, helping to bolster the reliability of your findings.
Using Margin of Error and Statistical Analysis for Confidence
In conducting reliable statistical analysis, integration of margin of error allows a clearer understanding of confidence intervals. When hypotheses are confirmed with a narrow margin of error, decision-makers can be more confident in basing their strategies on these findings.
Real-world example: If a recent survey's results show a significant increase in customer satisfaction ratings from 70% to 75% with a margin of error of ±3%, the data indicates a confidence level that supports the conclusion that the increase is meaningful rather than coincidental. This translates to more confident predictions and informed decision-making processes.
Conclusion: Practical Applications and the Future of Data Analysis
As we conclude this guide on calculating margin of error, it is crucial to recognize its application across various fields, from research and data analysis to more extensive statistical evaluations. Mastering the techniques on how to calculate margin of error effectively leads to enhanced data accuracy, significantly improving the reliability of research findings.
As statistical methods evolve in 2025 and beyond, keeping abreast of best practices will be paramount. The ability to accurately assess results can empower researchers to make informed decisions, refine methodologies, and boost the credibility of their analysis.
In summary:
- Understand the implications of margin of error in effective survey results.
- Use proper sample size calculations to reduce error margin.
- Recognize the interplay between statistical significance and error margin.
- Continuously refine your statistical skills to adapt to evolving research methodologies.
By implementing these strategies, you will contribute to the future of data interpretation and harness the power of statistical analysis in decision-making.